This is a Preprint and has not been peer reviewed. The published version of this Preprint is available: https://doi.org/10.1002/jrsm.1663. This is version 3 of this Preprint.
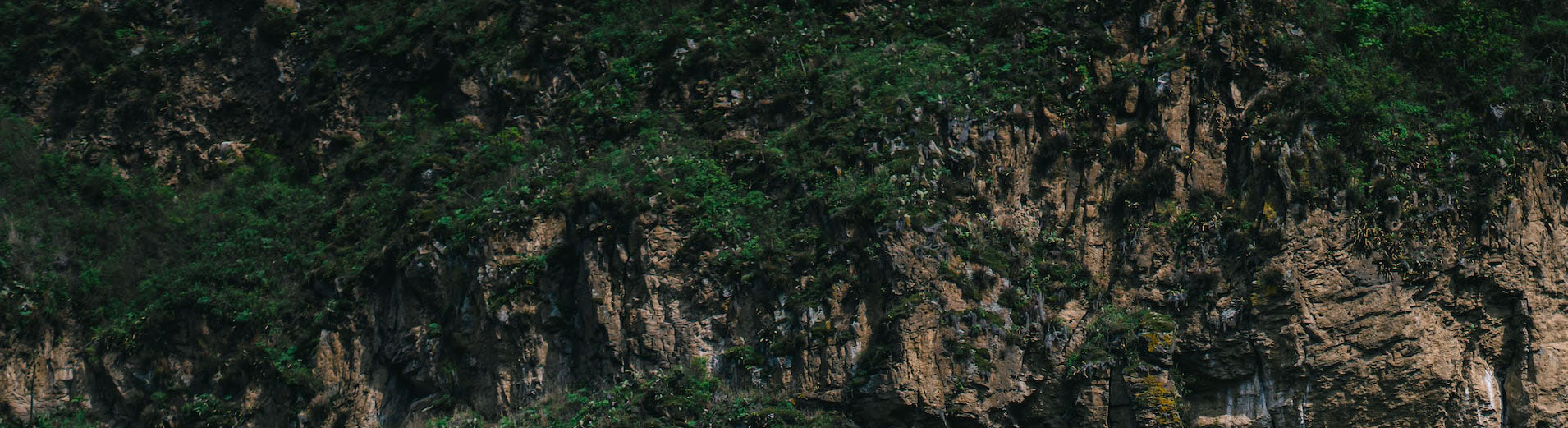
Downloads
Authors
Abstract
Extracting data from studies is the norm in meta-analyses, enabling researchers to generate effect sizes when raw data are otherwise not available. While there has been a general push for increased reproducibility in meta-analysis, the transparency and reproducibility of the data extraction phase is still lagging behind. Unfortunately, there is little guidance of how to make this process more transparent and shareable. To address this, we provide several steps to help increase the reproducibility of data extraction in meta-analysis. We also provide suggestions of R software that can further help with reproducible data policies: the shinyDigitise and juicr packages. Adopting the guiding principles listed here and using the appropriate software will provide a more transparent form of data extraction in meta-analyses.
DOI
https://doi.org/10.32942/X2D30C
Subjects
Ecology and Evolutionary Biology, Life Sciences, Psychology, Research Methods in Life Sciences
Keywords
meta-analysis, data extraction, shiny, metaDigitise, reproducibility, data extraction, metaDigitise, juicr, shinyDigitise
Dates
Published: 2022-12-14 10:29
Last Updated: 2023-08-15 16:59
Older Versions
License
CC-By Attribution-NonCommercial-NoDerivatives 4.0 International
Additional Metadata
Conflict of interest statement:
The authors declare no conflict of interest.
Data and Code Availability Statement:
Data and code for the metaDigitise analysis can be found here: https://github.com/EIvimeyCook/DataExtraction_MS
There are no comments or no comments have been made public for this article.