This is a Preprint and has not been peer reviewed. The published version of this Preprint is available: https://doi.org/10.1111/2041-210X.13352. This is version 1 of this Preprint.
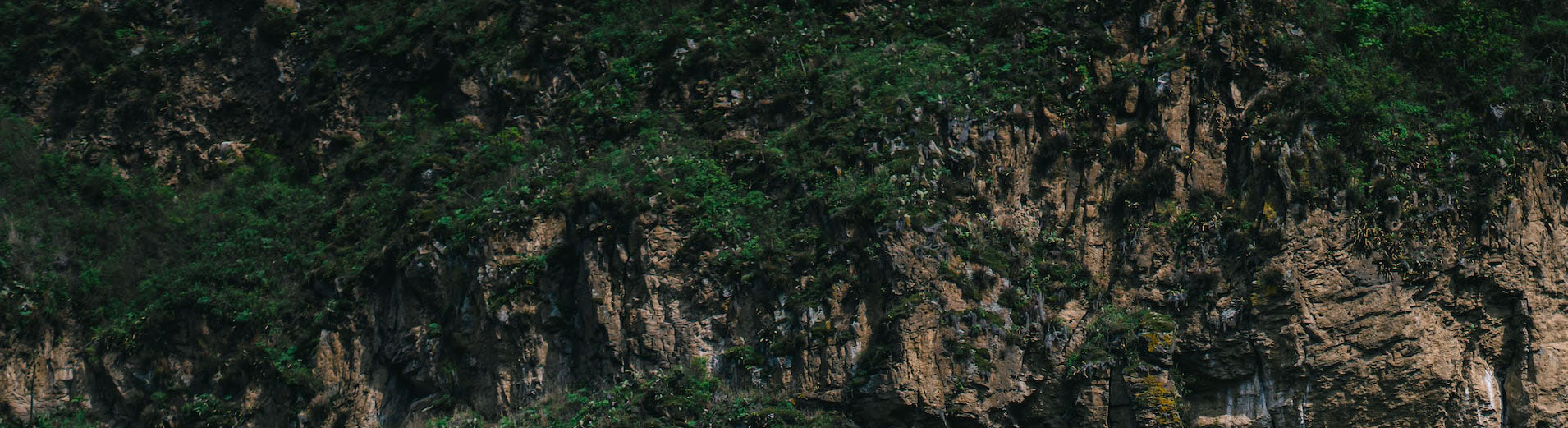
Slicing: a sustainable approach to the analysis of long-term biobanks
Downloads
Authors
Abstract
The longitudinal study of populations is a core tool for understanding ecological and evolutionary processes. These studies typically collect samples over individual lifetimes and across multiple generations, building up a continuously growing biobank from which samples are then analysed in clusters over time in the laboratory. To ensure data are comparable among clusters we need to account for among-cluster variation and confounding variables, yet this is often ignored.
The commonly used approaches in structuring samples for analysis, sequential and randomisation, generate bias due to non-independence between their time of collection and cluster. We propose a new sample selection strategy, slicing, specifically designed to statistically account for this bias. Slicing would, however, be suboptimal if aggregating longitudinal samples of the same individual within a single batch reduces measurement error and thereby increases statistical power to detect within-individual effects, a notion we challenge using simulations.
Our slicing approach, whereby recently and previously collected samples are analysed in a cluster together, enables statistical separation of collection time and cluster effects through appropriate mixed models. Additionally, we recommend the use of internal controls (reference samples) to further assess among-cluster variation. Our simulations show similar precision and higher statistical power to detect cohort, within- and between-individual effects when samples are sliced across batches, compared with strategies that aggregate longitudinal samples or use randomised allocation.
While the best approach to analysing long-term datasets depends on the structure of the data and questions of interest, it is vital to account for among-cluster and batch variation. This can be achieved through mixed models and appropriate sample selection strategies. Our slicing approach is simple to apply and creates the necessary statistical independence of batch and cluster from environmental or biological variables of interest. Crucially, it allows subsequent samples to be added in later analyses without completely confounding them with cluster. Our approach maximises the value of every sample, as each will optimally contribute to unbiased statistical inference from the data. Slicing therefore has the potential to maximise the power of growing biobanks to address important ecological, epidemiological and evolutionary questions.
DOI
https://doi.org/10.32942/osf.io/u3krn
Subjects
Life Sciences, Research Methods in Life Sciences
Keywords
Ageing, biobank, internal controls, longitudinal, long-term studies, mixed models, slicing, telomeres
Dates
Published: 2018-12-19 08:56
There are no comments or no comments have been made public for this article.