This is a Preprint and has not been peer reviewed. The published version of this Preprint is available: https://doi.org/10.1080/13658816.2021.1893324. This is version 1 of this Preprint.
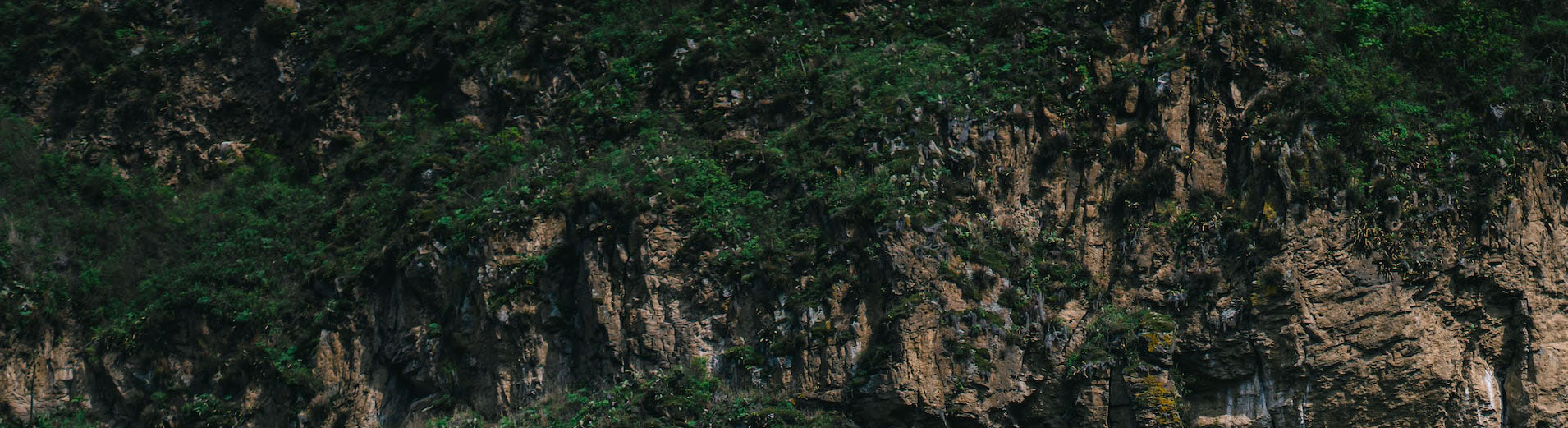
Downloads
Supplementary Files
Authors
Abstract
Categorical maps of landscape types (LTs) are useful abstractions that simplify spatial and thematic complexity of natural landscapes, thus facilitating land resources management. A local landscape arises from a fusion of patterns of natural themes (such as land cover, landforms, etc.), which makes an unsupervised identification and mapping of LTs difficult. This paper introduces the integrated co-occurrence matrix (INCOMA) – a signature for numerical representation of multi-thematic categorical patterns. INCOMA enables an unsupervised identification and mapping of LTs. The region is tessellated into a large number of local landscapes – patterns of themes over small square-shaped neighborhoods. With local landscapes represented by INCOMA signatures and with dissimilarities between local landscapes calculated using the Jensen-Shannon Divergence (JSD), LTs can be identified and mapped using standard clustering or segmentation techniques. Resultant LTs are typically heterogeneous with respect to categories of contributing themes reflecting the human perception of a landscape. LTs calculated by INCOMA are more faithful abstractions of actual landscapes than LTs obtained by the current method of choice – the map overlay. The concept of INCOMA is described, and its application is demonstrated by an unsupervised mapping of LT zones in Europe based on combined patterns of land cover and landforms.
DOI
https://doi.org/10.32942/osf.io/nzhu4
Subjects
Earth Sciences, Environmental Sciences, Physical Sciences and Mathematics
Keywords
global categorical datasets, pattern similarity, regionalization, spatial patterns, thematic maps
Dates
Published: 2022-03-06 15:15
There are no comments or no comments have been made public for this article.