This is a Preprint and has not been peer reviewed. The published version of this Preprint is available: https://doi.org/10.1111/ddi.13698. This is version 1 of this Preprint.
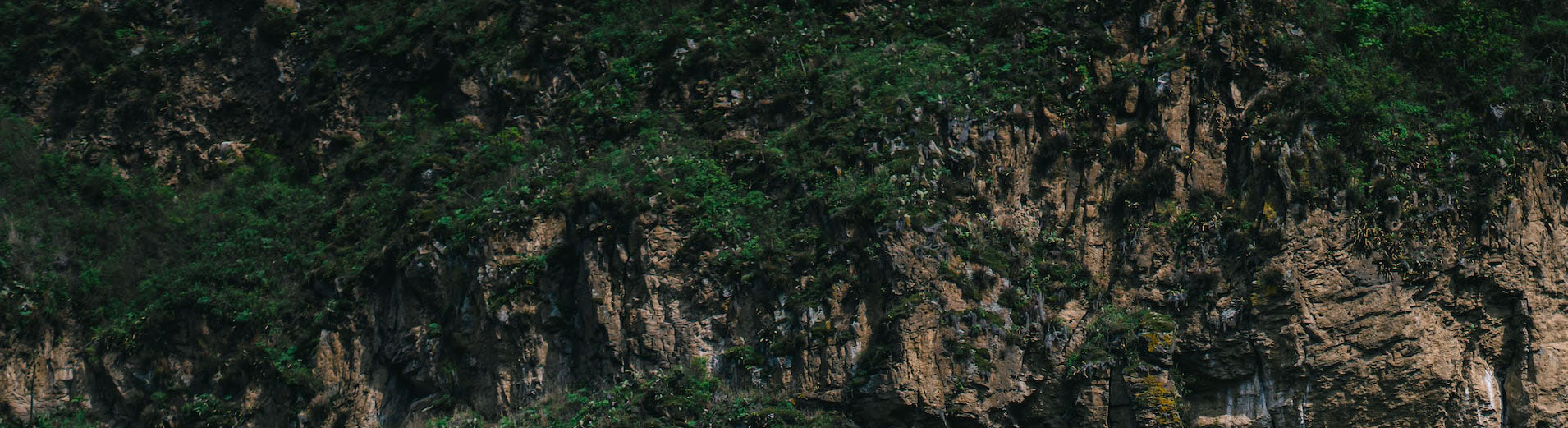
Causal inference and large-scale expert validation shed light on the drivers of SDM accuracy and variance
Downloads
Supplementary Files
Authors
Abstract
1. The literature is awash with studies purporting to show how various species and data characteristics affect the performances of Species Distribution Models (SDMs). Many of these studies follow a similar template: they fit SDMs for several species, or the same species using different datasets; assess the accuracy of those SDMs using skill statistics; and then identify correlates thereof. Interpreting the findings of these studies is challenging because skill statistics can reflect species and data characteristics rather than model accuracy, and correlates of model performance are not necessarily causes.
2. Here, we took a different approach to identifying causes of variation in SDM performance. We fitted models for 535 species across 5 invertebrate groups and 1 plant group in the United Kingdom (UK), using a fairly typical SDM workflow. We measured two components of SDM performance: the variance among replicate fits and accuracy. Rather than using skill statistics, accuracy was assessed by taxon experts. We constructed Directed Acyclic Graphs (DAGs) depicting plausible effects of explanatory variables (e.g. species’ prevalence, sample size) on SDM performance, then quantified those effects using multilevel piecewise path models.
3. We found that the degree to which the available data covered species’ environmental niches was the only explanatory variable to affect SDM accuracy. We suggest that previously reported associations between sample size and SDM accuracy reflect improved coverage of species environmental niches at higher sample sizes; that is to say, niche completeness confounds the effect of sample size on SDM accuracy. We also report that the completeness of species’ environmental niches, sample size, species’ prevalence and the degree to which the available data cover species’ geographic ranges affect SDM variance.
4. Our results demonstrate the challenges associated with the high-throughput approach to modelling species’ distributions. There is no guarantee that accurate and precise SDMs can be constructed for large numbers of species unless their ranges and niches have been sampled comprehensively. Decisions about whether modelling is worthwhile should not be based on simple criteria like sample size.
DOI
https://doi.org/10.32942/osf.io/68ats
Subjects
Biodiversity, Life Sciences
Keywords
Dates
Published: 2022-09-22 04:19
License
CC-By Attribution-NonCommercial-NoDerivatives 4.0 International
Additional Metadata
Data and Code Availability Statement:
The ensemble habitat suitability surfaces are embargoed until March 4th 2023, at which point they will become available at https://doi.org/10.5285/ec921bc2-5538-47ed-9e72-0d687b4ca4d3. We will provide the expert scores for these models when this article is accepted for publication.
There are no comments or no comments have been made public for this article.