This is a Preprint and has not been peer reviewed. The published version of this Preprint is available: https://doi.org/10.1016/j.ecoinf.2023.102391. This is version 1 of this Preprint.
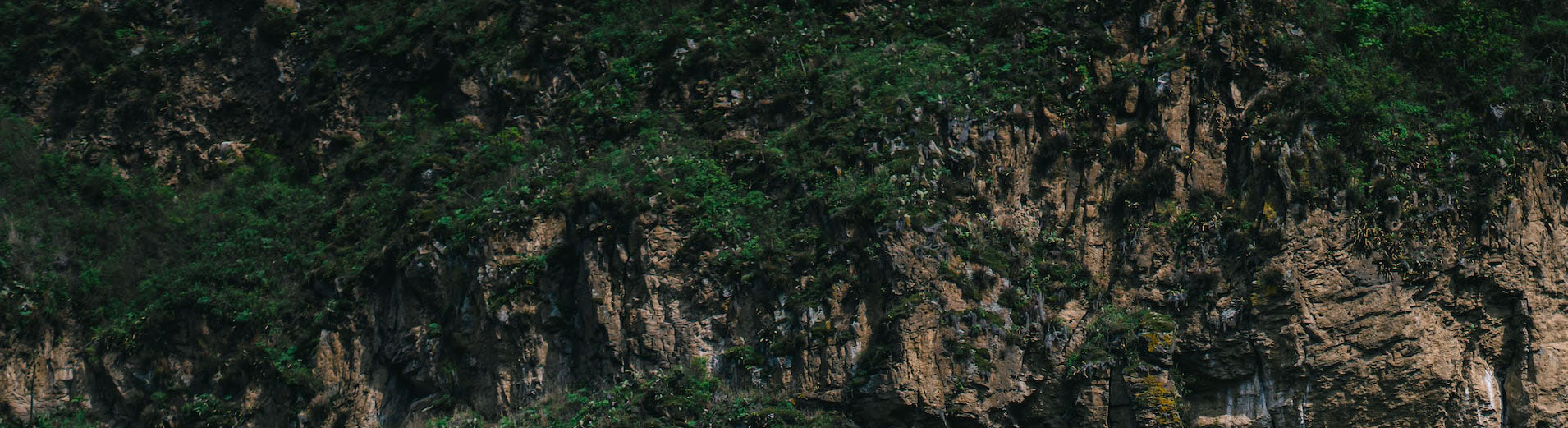
GridDER: Grid Detection and Evaluation in R
Downloads
Authors
Abstract
Observations and collections of organisms form the basis of our understanding of Earth’s biodiversity and are an indispensable resource for global change studies. Geographic information is key, serving as the link between organisms and the environments they reside in. However, the geographic information associated with these records is often inaccurate, thus limiting their efficacy for research. Along these lines multiple solutions for identifying erroneous coordinate data and records georeferenced to centroids or landmarks have been developed. Another prominent, but less discussed and documented source of inaccuracies arises due to the use of gridded survey systems in many regions of the world. Here we present GridDER, a tool for identifying biodiversity records that have been designated locations on widely used grid systems. Our tool also estimates the degree of environmental heterogeneity associated with grid systems, allowing users to make informed decisions about how to use such occurrence data in global change studies. We show that a significant proportion (~13.5%; 261 million) of records on GBIF, largest aggregator of natural history collection data, are potentially gridded data, and demonstrate that our tool can reliably identify such records and quantify the associated uncertainties. GridDER can serve as a tool to not only screen for gridded points, but to quantify the geographic and environmental uncertainties associated with these records, which can be used to inform models and analyses that utilize these data, including those pertaining to global change.
DOI
https://doi.org/10.32942/osf.io/6qy5u
Subjects
Biodiversity, Ecology and Evolutionary Biology, Environmental Sciences, Life Sciences, Physical Sciences and Mathematics
Keywords
Dates
Published: 2022-05-23 14:55
There are no comments or no comments have been made public for this article.